AI-driven drug discovery
How Iambic Therapeutics is Advancing Novel AI-Discovered Therapies with Help from Lambda Cloud
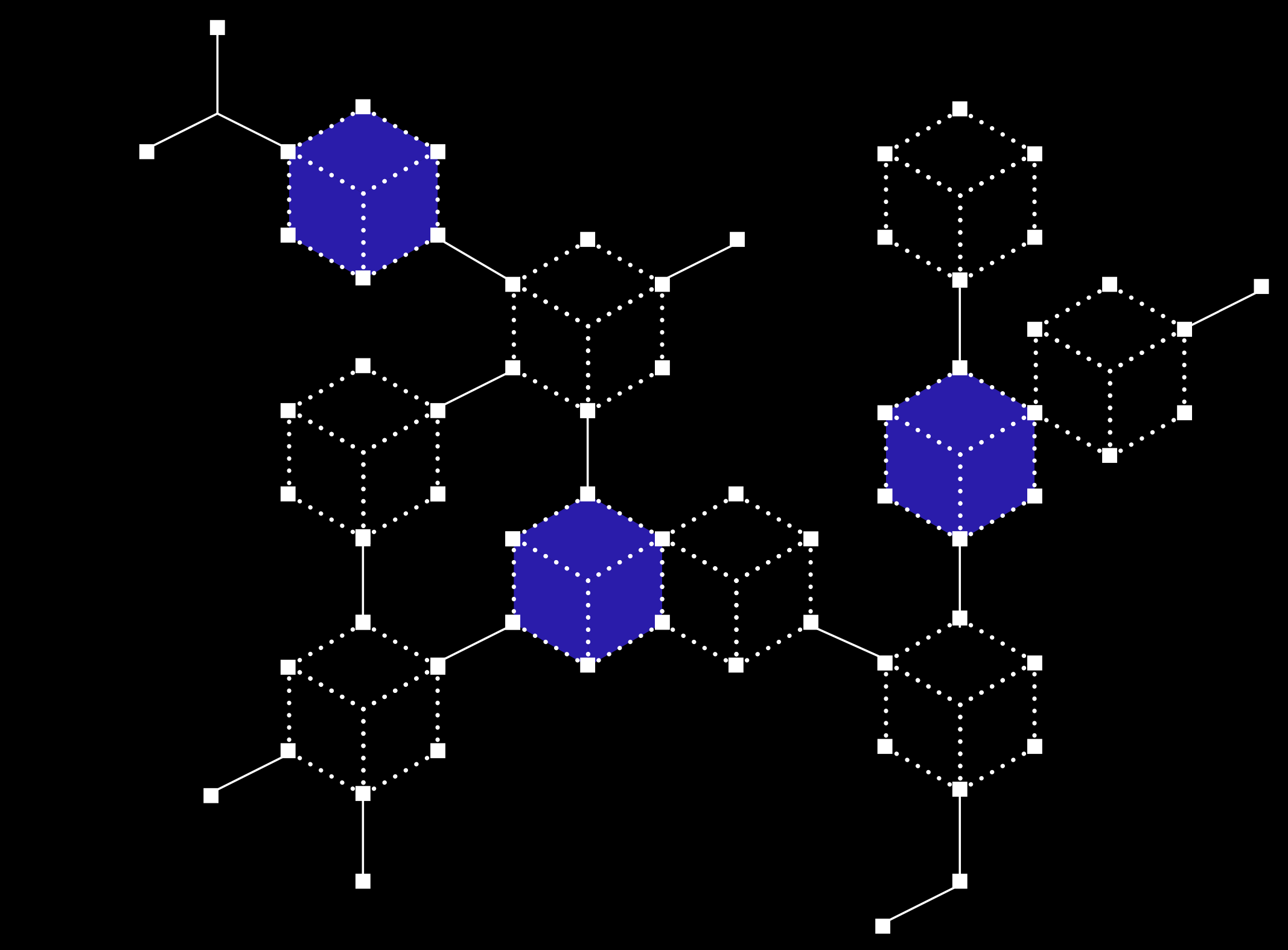
Customer
Iambic Therapeutics
Vertical
Drug Discovery
Products
1-Click Cluster
Challenge
AI presents exciting possibilities across industries, particularly in drug discovery, where AI has the potential to change lives, transform health outcomes, and improve the quality of medicine and the pace at which they are developed. Innovation in the field relies on the ability to access and train models on massive amounts of data from a vast array of sources. Recent developments have seen researchers gain the ability to use AI/ML tools to predict the structure of proteins, virtually on demand, while profoundly expanding their ability to search for novel chemical mechanisms that might lead to new and better medicines. The paradigm of discovery based on traditional laboratory methods is rapidly being complemented by in silico platforms that have the potential to increase the speed and decrease the cost of bringing new medicines to patients.
As models are trained on troves of preclinical data and those from publicly available sources, “the amount of data available that directly answers the question: ‘does this drug cure this disease’ is incredibly small,” says Matt Welborn, PhD., Iambic’s Vice President of Machine Learning. To help address the issue of data scarcity, Iambic employed longer training runs and more sophisticated model architectures to maximize available data, which in turn increased demand on their entire training stack.
This approach required immense computing power and the ability to scale across multiple GPUs with fast interconnects in order to train the models and rapidly experiment. Iambic explored working with hyperscalers but faced limitations—they needed reservations to access GPUs without wait times and additional labor to calibrate setup for specific use cases. The approach was too time-consuming, and Welborn estimated the need for a full-time person—40 hours a week, all year long—just to manage resource provisioning. Needing an agile partner, Iambic Therapeutics turned to Lambda.
Solution
How Lambda delivered on Iambic Therapeutics’ need for stable, agile compute
To build a system spanning experimentation to deployment that was both stable and scalable — and that also gave researchers the agility needed to iterate quickly — Iambic selected Lambda Cloud and the access it provided to NVIDIA H100 GPUs. Lambda’s deep AI expertise and round-the-clock service supports Iambic’s ongoing innovation, and as a cloud provider specializing in AI, Lambda has the knowledge and experience to address the broad needs of Iambic’s team. This included offering pre- configured infrastructure to support training and inference, enabling Iambic to focus on accelerating development of their state-of-the- art models. Additionally, to mitigate faults prevalent in more bare metal systems, Lambda configured a managed Kubernetes cluster.
Lambda also provides NVIDIA accelerated computing platform support for Iambic on a dedicated cluster, simplifying deployments and testing across model types.
To help address the issue of data scarcity, Iambic employed longer training runs and more sophisticated model architectures to maximize available data, which in turn increased demand on their entire training stack.
This approach required immense computing power and the ability to scale across multiple GPUs with fast interconnects in order to train the models and rapidly experiment. Iambic explored working with hyperscalers but faced limitations—they needed reservations to access GPUs without wait times and additional labor to calibrate setup for specific use cases. The approach was too time-consuming, and Welborn estimated the need for a full-time person—40 hours a week, all year long—just to manage resource provisioning. Needing an agile partner, Iambic Therapeutics turned to Lambda.
-
“Lambda is proactive in working with us to navigate how best to make use of Kubernetes clusters for high performance computing.”
Matt Welborn, PhD.
Vice President of Machine Learning
-
“Having one cluster that does it all and is built to support all of our use cases is of critical importance...we now only have to train people how to use one highly functional system.”
Matt Welborn, PhD.
Vice President of Machine Learning
-
“Lambda is available on Slack to solve matters and to work with us – often solving them in real time or on the same day.”
Matt Welborn, PhD.
Vice President of Machine Learning
Results
More effective models, built on more stable infrastructure, to accelerate the pace of drug discovery
Through this partnership, Iambic has, in part, trained two of its leading models on Lambda’s infrastructure – NeuralPLexer3 and EnchantTM. NeuralPLexer3 is the state-of-the-art model for predicting the structure of protein-ligand complexes and is a major advance in defining structural enablement in drug discovery. NP3 provides instant, accurate structural insights across the full range of protein classes and drug molecules enabling Iambic's scientists to explore therapeutic hypotheses at unprecedented speed and scale.
-
“ The scale and stability of the Lambda cluster, accelerated by NVIDIA, helped enable us to train NeuralPLexer3. We would not have trained as large of a model if we had to use less stable, less scalable systems.”
Matt Welborn, PhD.
Vice President of Machine Learning
Iambic's EnchantTM is a multi-modal transformer model designed to predict the clinical properties of molecules during early discovery, enabling researchers to understand the viability of a potential new drug before it enters human studies. EnchantTM uses abundant discovery- stage data, along with small amounts of human data, to predict clinical endpoints.
In doing so, EnchantTM may reduce the time and expense of drug development, as well as the impact on patients in clinical studies.
Lambda Cloud has proven itself to be a production-ready environment with an incredibly low rate of failure compared with other providers considered. Welborn noted there were only two node failures in six months working with Lambda —which was 97 percent less frequent than even the lowest average node outage rate of other providers, as reported by Reika.